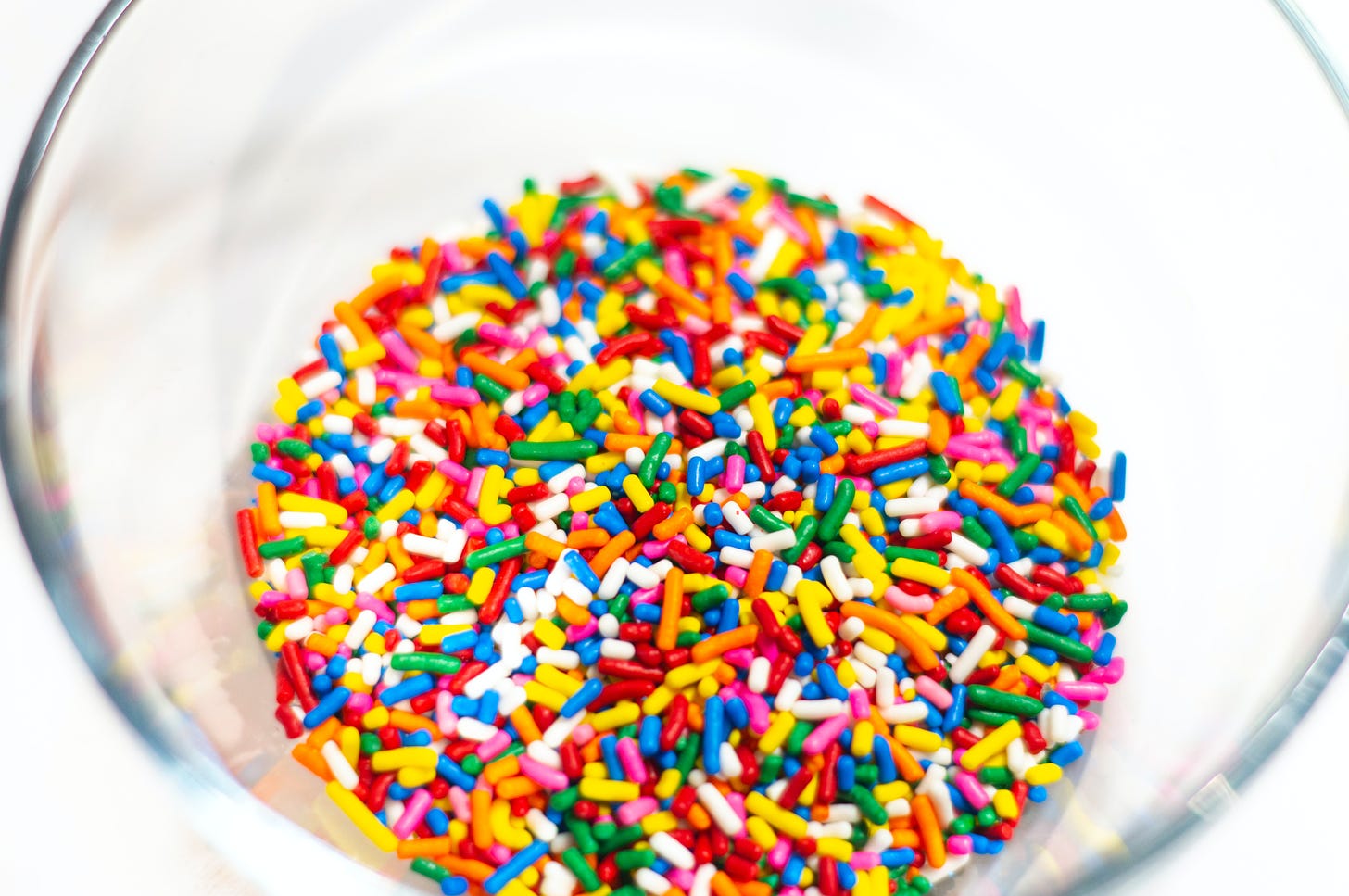
We all love frozen yogurt. Pinkberry took the froyo shop through a renaissance in the 21st century. I’ll tell you what I enjoy most about frozen yogurt.
I like it because it’s seemingly self service. I have the power to not only choose a flavor (or three) but also sample as many as I need to based on the descriptions above evenly spaced out levers. If I pull the lever that’s labeled chocolate, I’m fairly certain vanilla isn’t going to come out. After picking my flavors, I see all the toppings are organized and of course also labeled. I can take one spoonful of m&ms, two spoonfuls of sprinkles, and throw in a strawberry or two (gummy bears as a topping is anarchy, I said what I said).
To pay, the scale is ready at the register to weigh the froyo bowl I’ve put together and the price is equally clear from the start.
Self-service is the illusion of being able to make choices, while having the choices clearly explained and laid out.
If I told a friend we’re going to get froyo and brought them to a farm where they are expected to milk a cow, process the milk into yogurt, wait a few hours until it’s frozen and then flavor it, I’d look crazy.
But why do the stakeholders of analytics teams express a desire to see raw data that isn’t cleaned in any way, shape, or form?
Chaos in the data farm
Consider this farm scenario, where all stakeholders get access to raw data. A product team is often concerned with drop off points in the user experience. An implementation of event tracking tools like Amplitude, Segment, or Rudderstack generates the data to answer these types of questions.
A classic e-commerce flow consists of a user searching something, viewing a few products, carting the products of interest, entering shipping and billing information, then completing the order. Conceptually there are several possible reasons for drop-off: the user searched for something but didn’t find products of interest, either because they don’t exist or because the search functionality isn’t working well; the user viewed products but then didn’t cart any of them because they’re too expensive; the user got to the point of entering their information, but maybe something was unclear on that page and they couldn’t complete the order. These are only speculative—the point is, the first step is figuring out where most users drop off. Is it after search, or right before completing the order?
To answer that question, there are many different ways to do user funnel analysis. All of them, however, assume that events fire when they are supposed to fire and contain all the relevant information needed.
What would happen if that ProductCarted
event also fires when a user views a product? This is obviously unintentional, but would make it seem like every person that viewed a product also carted it, when in reality it could be a big drop-off point. Alternatively, it would be reasonable that the two pages where first a user needs to enter their shipping address and second enter their credit card both fired a CheckoutPageView
event that has a page
property containing either address
or credit_card
to differentiate between the screens. What if that property field was empty 20% of the time? Either 20% of the data for funnel analysis would be missing, or the event is also firing outside of those two screens. Either way, the funnel analysis wouldn’t be fully understood.
The issues I outlined are purely testing issues. But what about the context of web analytics overall? Some small but not insignificant percentage of users isn’t going to have events at all because they use ad-blockers. Another slightly larger but not insignificant percentage of users might be duplicated depending on when in the flow they login as opposed to the backend relying on cookies to identify people. Where in the raw data does this context get articulated?
Stakeholders are ready to draw insights. This can be a great thing, but can work against them in a framework where raw data is exposed, does not have necessary context, and the proper testing frameworks aren’t in place.
Reduce skepticism: testing and context building
Data people are naturally skeptics, so a 100% conversion rate wouldn’t fly. However, stakeholders are inherently biased and don’t have their data quality hats on, nor should they.
When I go into a froyo shop, I’m not skeptical of pulling the chocolate lever and not knowing what’s going to come out. If I was, I would just find a different place to get froyo quite honestly. Similarly, stakeholders shouldn’t need to be skeptical—the skepticism should be handled upstream and they should get all the context they need.
Dashboards aren’t going to be enough for many stakeholders, but data democratization doesn’t start at data discovery—it starts at data testing and context building.
Step 1: Test before shipping to make raw data not so raw.
There are many tools out there for traditional data quality, but web analytics is a different beast. Specific event tracking tools have their own solutions like Segment Protocols, but a more holistic solution would be using tools like Avo. Manual testing is painful, and humans are prone to error. Making raw data not so raw by setting expectations for web events is one way to reduce skepticism.
Step 2: Reduce the need for context be democratizing metrics instead of data.
Metrics should be defined in one place and referenced by someone with both the analytics and business context to connect data to real user behavior. For instance, a metric on the percent of users that searched for products should have a denominator including all users that have events, not necessarily all users ever.
Finally, democratize and prosper.
Data literacy doesn’t go away
Democratization by itself will never be enough. Documentation is important for building context.
Data literacy can always be increased, and this leads to discussing organizational structure and centralization which is another topic in itself. Literacy includes context as well as reducing bias when reading data, leading to stakeholders accepting the data that doesn’t lead them to the answer they want.
In the meantime, I’ll be eating my popcorn watching metrics tools battle it out over market share.
Thanks for reading! I love talking data stacks so please reach out. I’d love to hear from you.
Hi Sarah, I came across your talk on the Databand site and I was wondering if you had time for a brief chat about the company and industry in general. I can provide more details in another channel. I also reached out to you via LinkedIn. My link is here: https://www.linkedin.com/in/craig-brett-creative/
Thank you.
Craig Brett